Comparison Methods For Stochastic Models And Risks
Posted : admin On 02.09.2019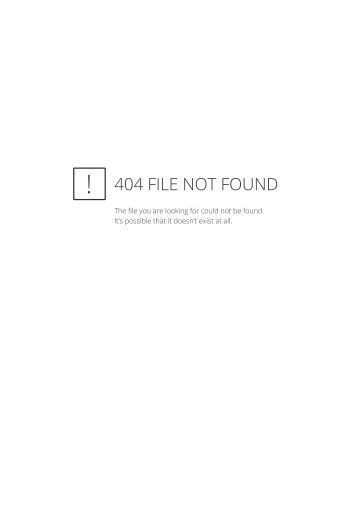

Stochastic Environmental Risk Assessment
Other hand, stochastic models and theory have evidently developed in the last 20 years. Warehouse practitioners and researchers need suitable methods to research warehouse problems in a stochastic environment. Stochastic models may help understand the impact of stochastic factors on the operational processes and system performance.
Stochastic Risk Analysis
- Abu-Amsha, O., Vincent, J.M.: An algorithm to bound functionals of Markov chains with large state space. In: 4th INFORMS Conference on Telecommunications, Boca Raton, Florida (1998)Google Scholar
- Benmammoun, M., Busic, A., Fourneau, J.M., Pekergin, N.: Increasing convex monotone Markov chains: theory, algorithms and applications. In: Markov Anniversary Meeting, pp. 189–210. Boson Books (2006)Google Scholar
- Busic, A., Fourneau, J.M.: Bounds for Point and Steady-State Availability: An Algorithmic Approach Based on Lumpability and Stochastic Ordering. In: Bravetti, M., Kloul, L., Zavattaro, G. (eds.) EPEW, WS-FM 2005. LNCS, vol. 3670, pp. 94–108. Springer, Heidelberg (2005)Google Scholar
- Courtois, P., Semal, P.: Bounds for the positive eigenvectors of nonnegative matrices and for their approximations by decomposition. J. of ACM 31, 804–825 (1984)zbMATHCrossRefMathSciNetGoogle Scholar
- Dayar, T., Fourneau, J.M., Pekergin, N.: Transforming stochastic matrices for stochastic comparison with the st-order. RAIRO-RO 37, 85–97 (2003)zbMATHCrossRefMathSciNetGoogle Scholar
- Dayar, T., Pekergin, N., Younes, S.: Conditional Steady-State Bounds for a Subset of States in Markov Chains. In: SMCTools, Pisa, Italy (2006)Google Scholar
- Fourneau, J.M., Le Coz, M., Quessette, F.: Algorithms for an irreducible and lumpable strong stochastic bound. Linear Algebra and Applications 386, 167–186 (2004)zbMATHCrossRefMathSciNetGoogle Scholar
- Fourneau, J.M., Le Coz, M., Pekergin, N., Quessette, F.: An open tool to compute stochastic bounds on steady-state distributions and rewards. In: IEEE Mascots 2003, Orlando, USA (2003)Google Scholar
- Fourneau, J.M., Pekergin, N.: An algorithmic approach to stochastic bounds. In: Calzarossa, M.C., Tucci, S. (eds.) Performance 2002. LNCS, vol. 2459, pp. 64–88. Springer, Heidelberg (2002)CrossRefGoogle Scholar
- Haddad, S., Moreaux, P.: Sub-stochastic matrix analysis for bounds computation-Theoretical results. Eur. Jour. of Operational. Res. 176, 999–1015 (2007)zbMATHCrossRefMathSciNetGoogle Scholar
- Meyer, C.D.: Stochastic complementation, uncoupling Markov chains, and the theory of nearly reducible systems. SIAM Review 31(2), 240–272 (1989)zbMATHCrossRefMathSciNetGoogle Scholar
- Meyer, C.D.: Matrix Analysis and Applied Linear Algebra. SIAM (2000)Google Scholar
- Pekergin, N., Dayar, T., Alparslan, D.: Compenent-wise bounds for nearly completely decomposable Markov chains using stochastic comparison and reordering. Eur. Jour. of Op. Res. 165, 810–825 (2005)zbMATHCrossRefMathSciNetGoogle Scholar
- Muller, A., Stoyan, D.: Comparison Methods for Stochastic Models and Risks. Wiley, New York (2002)Google Scholar
- Shaked, M., Shantikumar, J.G.: Stochastic Orders and Their Applications. Academic Press, San Diago (1994)zbMATHGoogle Scholar
- Trivedi, K.S.: Probability and Statistic with Reliability, Queueing and Computer Science Applications. Second Edition, Wiley (2002)Google Scholar
- Truffet, L.: Near Complete Decomposability: Bounding the error by a Stochastic Comparison Method. App. Prob. 29, 830–855 (1997)zbMATHCrossRefMathSciNetGoogle Scholar
- Truffet, L.: Reduction Technique For Discrete Time Markov Chains on Totally Ordered State Space Using Stochastic Comparisons. Journal of Applied Probability 37(3) (2000)Google Scholar
- Zhao, Y.Q., Liu, D.: The Censored Markov chain and the Best Augmentation. Jour. of App. Prob. 33, 623–629 (1996)zbMATHCrossRefMathSciNetGoogle Scholar